Science & Technology
A Scholarly Quest in Statistics
By Ala Paruch and Lucy Wu
Volume 1 Issue 8
June 8, 2021
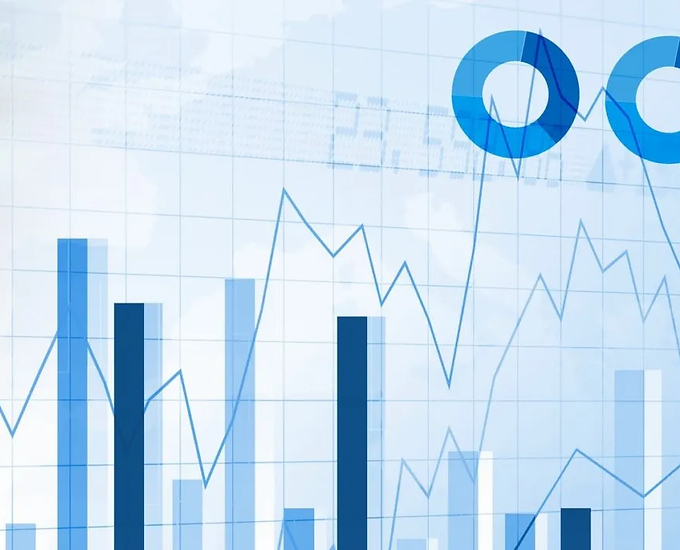
Image provided by the Corporate Finance Institute
With the rush of submitting the last few assignments and completing in-class finals for the school year, students were distraught and stressed. So rather than take a boring paper final, as per tradition, AP Statistics students were instead sent on a ‘quest’ to design, carry out, and present a research study or experiment of their choice.
Researching a Question
To begin our little ‘quest,’ we formulated our research question with the limitations of Covid in mind. In past years (aka the “before Covid”), students were permitted to conduct their study by going around the classrooms and asking students questions, or even asking them to taste test different foods. However, this year, we tried to avoid studies structured that way in the interest of time, but primarily in the interest of safety as well (I know, I know, we could have answered the age-old question of Pepsi vs. Coke, but I guess that’s another unknown in this world). As much as that seems like a disadvantage, it gave us a good opportunity to utilize Teams and send everyone their surveys digitally. Equipped with as much determination and motivation as juniors in a pandemic can have (to be fair, it’s dwindling quite quickly…) we are committed to making our project work, regardless of the treacherous obstacles in our way. This also sparked an idea for our research question. Since the start of quarantine, most people have been using technology more to stay connected. We assumed that to pass the time, most people have probably binge watched many TV shows (at least we have). So why not examine exactly that? In the end, we decided to research whether the grade of the student is associated with their favorite TV show.
Designing the Experiment (How do we do this?)
Once we determined our research inquiry, we had to finalize a few more details to continue our quest. First, we had to establish the explanatory and response variables in the study, or in other words, what causes (explanatory variable) the outcome of our study (response variable). Looking back at the question, we can safely say that the grade level affects the preference in the TV show, and not the other way around, so grade level is the explanatory variable and TV show preference is the response variable. Next, we had to formally establish the parameter of study (what we are studying) and the population of interest (who we are studying). Here we were looking at the favorite TV show (parameter of study) of all students at Valley Stream North from grades 7-12 (population of interest). Seems straight-forward so far, right? Well, let’s get into the nitty-gritty details - the experimental design. We had to establish a random method of collecting our data to fairly sample the population and get a representative sample. To equally represent each grade in our experiment, we chose English as the class we would send the survey to, since all students in the building are required to take English every year (and no, we didn’t want to randomly select from a roster because that would take an EXTREMELY long time to obtain all the names). Next, we established a system of selecting one class period per grade. Thanks to Ms. Small, we obtained a list of all English classes, along with their class period and name of the teacher. We then organized all classes by grade and assigned each class within the grade a number from 1 to n (n being the total number of English classes per grade). This process is also known as cluster sampling. Using the random integer generator function on the Ti-84 CE calculator (your trusty yellow Algebra I calculator), we randomly selected our sample classes, one class from each grade. Each student in each one of the selected classes is part of our sample. Upon choosing our sample, we expanded on the research question and formed a hypothesis, or rather the null and alternative hypotheses (those are fancy statistical words to define no statistically significant change vs. a statistically significant change - and don’t worry, we’ll cover what statistically significant means in a little bit).
Collecting Our Data (and now… we wait)
As stated before, we wanted to harness the power of Microsoft Forms to obtain our data for easy distribution and even easier analysis with Microsoft Excel (by the way, this article was NOT sponsored by Microsoft in any way, shape, or form). In fact, if you want to take a look, here’s the link to the original Microsoft Forms survey we used: https://forms.office.com/r/km5zdEekpz. We simply asked each student in our sample two questions: their grade level and TV show of preference from the list (we’re not perfect, so we will address this limitation later, but you can take a guess at what we probably should have done in the meantime). Luckily, we obtained 100 responses to the survey. Since it was a voluntary survey, we did not anticipate that all 140 students would fill it out, but we attempted to account for this by picking a slightly larger sample. By the way, shoutout to all of the amazing English teachers who took the time to share the survey with their students!
Significance Testing (sounds scarier than it is)
In statistics, to obtain “evidence” that something is either statistically significant or not, we can use a fancy math test known as a significance test (literally does what it says: it determines how likely an event is to occur by random chance in comparison to a claim). Since the data that we collected contains two distributions (a mathematical function that shows the possible values for a variable and how often they occur), the grade of a student and the favorite TV show of a student, and we are testing the association between them (NOT CAUSATION), we used a Chi-Square test to perform our calculations (FYI it’s pronounced KAI not CHAI). But alas, it’s never that simple, is it? There are three flavors of the Chi-Square tests: a Chi-Square test for Goodness of Fit, a Chi-Square test for homogeneity, and a Chi-Square test for independence, with each having different thresholds and conditions. Since we are going to have a single sample of data (not two samples) with multiple choices (not a single line of data), this fulfills the conditions to use the Chi-Square Test for independence, where you test whether two distributions are independent from each other as the name suggests. At this stage, we select our alpha value (commonly written as α), which just sets the level where we conclude that there is either evidence of significance or no evidence of significance. If the p-value (probability value, or how likely something is to occur) generated by our significance test is HIGHER than our alpha, there is no evidence that there is a significant difference, whereas if the p-value is lower than our alpha, there is statistical evidence that there is a significant difference. Since this is a non-life-threatening decision, we used the standard 0.05 threshold for our study.
After picking the alpha, there is one more task we must complete to perform the significance test, and that is ensuring that the conditions for the test are satisfied. The conditions for a Chi-Square Test for independence are as follows:
The Random Condition, which requires the researchers to obtain a sample randomly or to randomly assign a treatment to a group of volunteers. This checks whether bias occurred in the sampling process and helps eliminate it.
The 10% Condition, which uses the formula. 0.1N n (Where n in the sample size and N is the population of interest size). In simpler terms, this means that the sample we pull from the population has to be less than or equal to 10% of the size of the population of interest.
The Expected Counts Condition estimates the probability of each cell in a two-way table of a Chi-Square data set considering the null hypothesis is correct. In short, it calculates the estimated probability for each category in theory, which we later compare with real life results. However, this condition requires all values in the data set to be equal or greater than 5.
We fully met the random condition and and the 10 percent condition, because we randomly picked the class periods to fill out the survey, and it is safe to assume that there are more than 1000 students (n*10 = 100*10 = 1000) at Valley Stream North High School. On the other hand, the expected counts were not fully satisfied, with a few values below 5; however, we still continued our experiment, but continued it with caution.
Analyzing the Data
You know the drill now! First, we sorted and tallied the data by TV show preference for each of the grades. We input that table into a matrix in our calculator. Using the X2-Test (Chi-Square test) function on our handy calculators, we generated a p-value for our data. Place your bets now on whether the test was statistically significant or not, and no cheating! Drumroll please… with a p-value of 0.54 which was (drastically) higher than our alpha value of 0.05, the test was not statistically significant!
With this rather disappointing result, we fail to reject the null hypothesis that the tests are independent. We did not find sufficient evidence to conclude that there is a significant difference between the two distributions. Why use the confusing wording to formulate our conclusion you may ask? Well, let’s try to understand. The term “fail to reject” implies exactly what the second sentence of the conclusion said: we did not find enough evidence (or our p-value was nearly not small enough) to say that the null hypothesis was wrong. However, just because the null hypothesis isn’t incorrect, it doesn’t mean that it is correct and using statements such as “accept the null hypothesis” would mathematically be incorrect and just would also just be a statistical faux pas.
Discussing our Limitations (uh-oh…)
Sailing was smooth… until it wasn’t. So, we ran into some rough waters at certain stages of the process.
When designing the questions, we only realized when it was too late that we should have probably asked the questions in terms of TV show genre rather than specific shows, to decrease the likelihood of a student choosing “other” and inflating that category, compromising the integrity of our study.
We were pressed for time, so we had to rely on the integrity of the students (most of whom did very well) to answer the survey. Since this survey was a voluntary response survey, we could not control the nonresponse bias, so anyone who didn’t respond was sadly not part of the study.
There was also the risk of the teacher posting the survey for their class not responding. Since we decided to give out the survey digitally, we reached out to the teachers on Teams in hopes that they would be willing to post our survey on the teams for each designated class. With that arose the possibility of them not seeing the message or even humanly reading it and then not responding, besides the usual risk of them saying “no.” Thankfully, that didn’t happen; however, it was significantly harder to ensure we got any responses at all.
Lastly, (and perhaps most unfortunately), out of all the shows we anticipated would be popular among students, not a single student picked Jeopardy! as their favorite show to watch. Besides the shame this is, it also posed problems during the data analysis.
Finishing with Conclusions (Hooray! We did it.)
If you take anything away from this article, please remember: AP Statistics is the best class at Valley Stream North High School (and no, we received no extra points for saying that). We hope you will embark on the same journey we took and challenge your own perception of statistics.
Going back to formal conclusions though, as we mentioned earlier, we did not get a statistically significant result, so we can sadly admit that as of now, there is no clear association between a student’s grade level and their favorite TV show. Even though we didn’t get the exact results we were hoping for, there are still a few things we learned from this experience.
Firstly, we learned that designing an experiment is more (emotionally) draining than we initially thought.
We also learned how to design an experiment that is safe for the public amidst a pandemic.
Lastly, we tested the bounds of our friendsh- just kidding, we had a lovely time working with each other! We did spend long phone calls discussing the appropriate Chi-Square test to use, alongside collecting responses from many, many students. Yet because of this quest to design an experiment, our friendship only grew stronger with each statistical obstacle we overcame.
But even if things don’t work out (like our expected counts), remember to hold your head up high, triple-check your calculations, and just "keep swimming!